This post is part of G2's 2024 technology trends series. Read more about G2’s perspective on digital transformation trends in an introduction from Chris Voce, VP, market research, and additional coverage on trends identified by G2’s analysts.
Why generative AI will still fall short for enterprises in 2024
Prediction
In 2024, businesses of all sizes will use generative artificial intelligence (GAI), but enterprise adoption will lag other segments. GAI will mainly be used for content creation, not complex operations like manufacturing or supply chain.
Let's explore the reasons behind this and the challenges faced by generative AI to be truly enterprise ready.
The current state of enterprise generative AI
Generative AI encompasses a range of technologies, including AI chatbots and large language models (LLMs), that can create text, images, videos, and even code. Its potential applications span from content generation to healthcare diagnostics and autonomous vehicles.
To understand how this potential is currently fulfilled, I looked at G2 data to see how GAI is used in two categories: AI Chatbots Software (tools like ChatGPT to support end users) and Large Language Models (LLMs) Software (the engine behind AI chatbots).
Here's what I found:
1. Only 13% of AI chatbots reviewers work for companies with more than 1,000 employees, and the percentage of enterprise users for LLMs is even lower, at 8%. By comparison, 24% of ERP Systems reviewers work for enterprises.
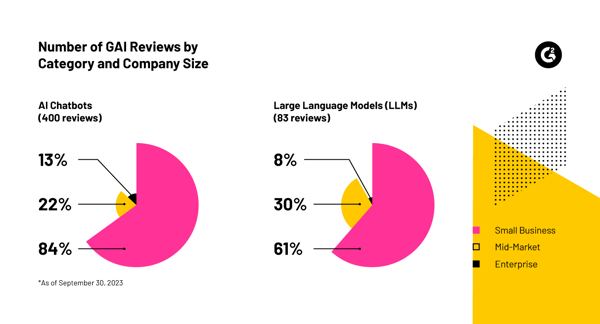
2. The top five industries of enterprise GAI users were significantly different by category, and most of them are related to professional services (with a few exceptions, such as apparel and automotive).
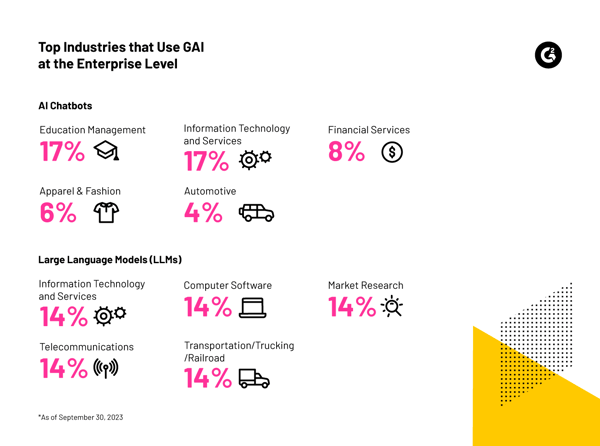
3. The main business benefits that enterprise GAI users realized were content and writing, coding and programming, business communication (emails and chat), and reporting.
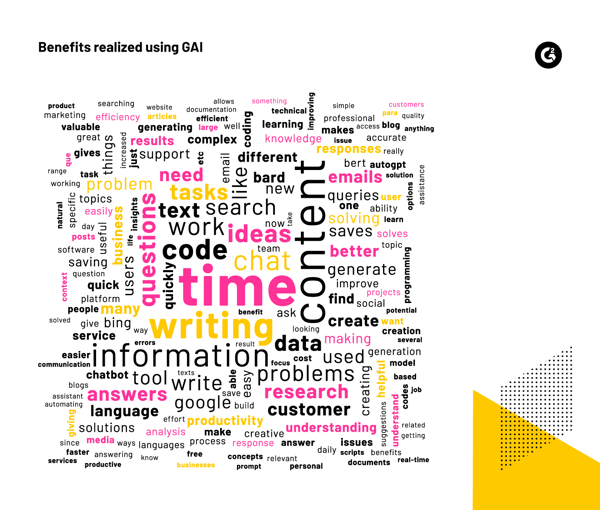
Not only does our data show that GAI adoption at the enterprise level is very low, but the use cases mentioned by enterprise reviewers refer mainly to content creation, not complex operations like manufacturing or supply chain.
Challenges in enterprise adoption of generative AI
While GAI is already being used successfully in large organizations like Boston Consulting Group (BCG), generative AI faces significant hurdles regarding enterprise adoption:
- Data privacy and security concerns: Enterprises deal with sensitive data, and using generative AI on this data raises significant privacy and security concerns. Protecting proprietary information and customer data becomes a challenge.
- Lack of interpretability and transparency: Enterprises require models that can explain their decisions. Generative AI often operates as a black box, making understanding its thought processes and results challenging.
- Scalability issues: Generative AI models are resource-intensive, demanding substantial computational power and large datasets. Scaling up to meet enterprise demands can strain infrastructure and budgets. Integrating these resource-hungry models into existing systems poses additional challenges.
Surpassing these challenges is feasible but requires tremendous efforts (financial and operational) from both buyers and sellers of GAI technology. It will also take time, probably years of trial and error.
How software vendors can make generative AI enterprise ready
The journey to making generative AI enterprise-ready is ongoing. Enterprises must carefully consider the challenges and weigh the benefits before embracing generative AI fully.
As for software vendors, they need to focus on the following to make GAI enterprise ready:
- Partnerships with other vendors and market data and intelligence providers, such as Lexis Nexis, Crunchbase, Dun & Bradstreet, or NASDAQ. Most enterprises use software from multiple vendors and external data sources, and GAI should be trained using all this data.
- Anonymizing sensitive business data while keeping enough valuable information to train LLMs. For instance, names or credit card numbers are unnecessary for financial analysis, but the transaction amount and frequency are critical.
- Customization, since enterprises have diverse requirements, and a one-size-fits-all approach doesn't suffice. Tailoring of generative AI to specific enterprise needs can be achieved through extensive development, testing, and refinement.
Enterprise generative AI: when will it be ready?
While generative AI holds immense promise, it currently faces significant obstacles to enterprise readiness. Addressing data privacy, ethics, scalability, and customization issues is essential for realizing its full potential in the corporate world.
The complexity of these challenges and the current low adoption rate will probably make enterprise-ready GAI a reality no sooner than 2025.
Confused about all the multiple terminologies related to generative AI? Dive into the different types of generative AI and associated technology.
Edited by Shanti S Nair